Reinforcement Learning
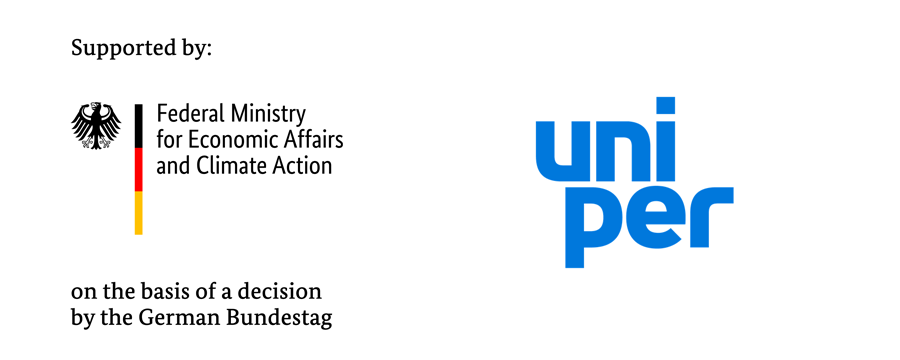
for controlling waste incineration plants
Many process engineering systems are nonlinear and also time-varying systems, which can often be controlled better by an experienced human operator than by a classical controller. The human ability to independently learn the correct behavior through interaction in an unknown environment is to be emulated by an algorithm. A reinforcement learning structure is suitable for this purpose, as it already outperforms human performance for many complex problems.
The interest in using these algorithms for controller synthesis is high and first implementations on simulated processes have already been carried out successfully. Now this concept is to be applied to real plants.
A municipal solid waste incineration plant (MSWI) is used as a pilot plant for this purpose. In the first step, a model is developed for this plant, which represents the dynamics and process fluctuations of the plant as realistically as possible. A hybrid model approach is pursued, in which neural networks are used for function approximation in addition to analytical differential equations.
Using this model, a neural network controller is to learn by trial-and-error how to control an MSWI in such a way that the optimal interventions are made in each operating state. The objective is to minimize a cost function in which control objectives can be defined and weighted. In the case of a waste incineration plant, this is, among other things, a constant steam production at a high waste throughput and low emissions. This method has the advantage that individual requirements can be intuitively introduced into the cost function and thus into the controller synthesis.
The project builds on very successful results already achieved by our industrial project partner Uniper SE in the development of a neural network for the control of a waste incineration plant.